Voice-to-text technology, often exemplified by services like Google’s, has revolutionized how we interact with devices and process information. From hands-free commands to automatically transcribing spoken words, its utility is undeniable. However, relying solely on automated voice transcription Google services, particularly for critical applications or nuanced languages like Japanese, presents significant challenges. Achieving perfect accuracy, handling complex linguistic structures, and ensuring data privacy are persistent hurdles. This is where services designed for precision and context come into play, helping bridge the gap between raw automated output and reliable documentation or translation.
The Problem: Accuracy and Nuance in Automated Voice Transcription
While advancements in AI and machine learning have drastically improved speech recognition, automated systems, including sophisticated ones like Google Cloud Speech-to-Text, are not without limitations. The primary challenge lies in achieving consistently high accuracy across diverse real-world conditions and linguistic complexities.
Expert insights reveal that factors such as background noise, the presence of multiple speakers, distinct regional dialects, slang, and unconventional phrasing can significantly reduce transcription accuracy. For the Japanese language specifically, the challenges are amplified by its unique grammatical structures, including frequent omission of subjects and objects, the wide variety of conjugations and particles, and a high prevalence of homophones. This makes accurate recognition inherently more difficult compared to some other languages, as highlighted in research on the mechanism and challenges of voice recognition technology by AI技術を活用した音声認識とは?仕組みや活用例、今後の課題まで – AI GIJIROKU and 音声認識の仕組みと課題丨音声をテキスト化する技術・アルゴリズムを解説|トラムシステム.
Furthermore, recognizing technical jargon, industry-specific abbreviations, company vernacular, and proper nouns not commonly found in standard language models poses another significant hurdle. As noted in an article discussing the usability of voice recognition, difficulty in recognizing industry-specific terms can be a problem, particularly in difficult audio environments. Even Google has acknowledged the challenge of capturing the nuances of Japanese dialects, though efforts are underway to improve accuracy through data learning, as discussed in an overview of Google Cloud Speech-to-Text by Google Cloud Speech-to-Textとは?基本的な概要と仕組み – 株式会社一創.
Beyond simple word recognition, automated systems often struggle with contextual understanding. The flexibility of Japanese grammar and its tendency to omit elements necessitates sophisticated processing to accurately infer meaning and produce a coherent transcript. Issues like handling multiple speakers and distinguishing voices amidst background noise remain persistent problems, impacting the reliability of transcriptions for meetings or interviews.
Finally, privacy concerns surrounding the use and storage of voice data are a valid consideration for users and organizations. While major providers like Google have implemented security measures and privacy filters, transparency about data handling practices is crucial for building trust, according to Google Cloud Speech-to-Textとは?基本的な概要と仕組み – 株式会社一創.
The Solution: Enhancing Accuracy and Reliability
Addressing the challenges of automated voice transcription requires a multi-faceted approach, combining technological advancements with strategic implementation. The core of the solution lies in improving the underlying models and incorporating methods to handle real-world complexities.
Improving accuracy necessitates the use of the latest acoustic and language models trained on vast, diverse datasets. Crucially, these datasets must include variations in accents, speaking styles, and domain-specific terminology. For Japanese, incorporating data that captures regional accents and subtle pronunciation variations is vital for enhancing recognition accuracy, as emphasized in research on improving Japanese voice recognition accuracy by 日本語音声認識の精度向上法|研究者必見の最新技術と事例 – Hakky Handbook.
Handling specialized vocabulary and jargon can be significantly improved by utilizing custom vocabulary and language models. Training these models on specific datasets relevant to an industry or use case allows the system to recognize technical terms and proper nouns with higher confidence. Google Cloud Speech-to-Text, for instance, offers custom model capabilities optimized for specific industries, which can improve accuracy for specialized terminology, according to Google Cloud Speech-to-Textとは?基本的な概要と仕組み – 株式会社一創.
Overcoming the challenges posed by noisy environments and multiple speakers requires advanced signal processing techniques. Noise reduction algorithms help filter out background sounds, while speaker diarization technology identifies who is speaking and when, producing a more organized and accurate transcript of multi-party conversations. Using high-quality microphones with built-in noise cancellation also contributes to cleaner audio input.
Enhancing contextual understanding in languages like Japanese demands more sophisticated Natural Language Processing (NLP) and the application of large language models (LLMs). These advanced models are better equipped to analyze sentence structure, infer missing elements, and understand nuances, leading to more coherent and contextually accurate transcriptions.
Finally, building user trust requires robust data security and privacy measures. Providers must be transparent about how voice data is collected, processed, and stored, implementing strong security protocols and offering privacy controls that comply with regulations. Google has taken steps to address these concerns by clarifying practices and implementing stronger security features, as noted by Google Cloud Speech-to-Textとは?基本的な概要と仕組み – 株式会社一創.
Implementation: Leveraging Technology for Accurate Transcription and Translation
Implementing effective solutions for voice transcription involves selecting the right tools and strategies for specific needs. While automated services like Google’s provide a powerful foundation, achieving high-quality, reliable results often requires additional steps, especially for critical tasks or when translation is involved.
For tasks where high accuracy is paramount, such as minute-taking for important meetings or transcribing interviews for research, relying solely on raw automated output may not be sufficient. Businesses in Japan are increasingly recognizing the value of voice recognition for improving efficiency, particularly in tasks like data entry and transcription, with predictions of the market nearly doubling by 2028, as noted by AI技術を活用した音声認識とは?仕組みや活用例、今後の課題まで – AI GIJIROKU. The adoption of AI-powered transcription and analysis is becoming more prevalent in business settings, includingコールセンター (call centers) and議事録作成 (minute taking).
One practical approach is to use automated transcription as a first pass and then employ human review and editing to refine the output. This hybrid approach combines the speed and scalability of automation with the accuracy and contextual understanding of human intelligence. For scenarios involving different languages or complex technical content, integrating translation services becomes essential.
This is where platforms like Doctranslate.io can add significant value. When dealing with transcripts from automated services, especially those containing nuances or specialized terminology that might challenge the initial voice-to-text process, ensuring the final document is accurate and reliable is critical. Doctranslate.io specializes in providing high-quality document translation that accounts for context, tone, and specific domain terminology, ensuring that the translated version of a transcript or meeting minutes is accurate and culturally appropriate. This is particularly relevant in the Japanese market, where the nuances of language can greatly impact business communication.
The future of voice recognition in Japan points towards continued advancements driven by AI and NLP, leading to more natural and accurate interactions. Integration into various sectors like smart homes, vehicles, and business applications will increase. A key trend is the focus on improving the recognition of Japanese-specific linguistic features and the use of generative AI for tasks like automatic summarization in applications like call centers to improve efficiency, according to insights from AI技術を活用した音声認識とは?仕組みや活用例、今後の課題まで – AI GIJIROKU.
Selecting tools that allow for customization, like using custom models for specialized vocabulary with services such as Google Cloud Speech-to-Text, is a valuable strategy. Furthermore, for critical audio, some suggest having a human operator repeat important information to improve recognition accuracy in challenging environments, according to 音声認識って本当に使えるの?と思っている皆さんへ | DIGITAL.
By understanding the limitations of automated voice transcription Google tools and implementing strategies such as combining automation with human oversight or leveraging specialized translation services for subsequent processing, organizations can achieve the accuracy and reliability required for professional use cases.
Conclusion
While Google’s voice-to-text capabilities are powerful and constantly improving, particularly with ongoing efforts to better handle languages like Japanese, challenges related to accuracy, noise, multiple speakers, specific vocabulary, and contextual understanding persist. Solutions involve leveraging advanced models, custom vocabularies, better signal processing, and enhanced NLP.
For businesses and individuals relying on voice transcription for important documents or requiring translation of spoken content, achieving high reliability is key. Simply using an automated service might not be enough. Future trends indicate increased market expansion for voice recognition in Japan and deeper integration into business processes, underscoring the growing need for accurate transcription and translation solutions.
To ensure your transcribed documents or translated content is accurate, contextually appropriate, and meets professional standards, consider solutions that go beyond basic automation. Services like Doctranslate.io provide expert document translation that can help refine or translate output from voice transcription, ensuring clarity and precision for your critical communication needs.
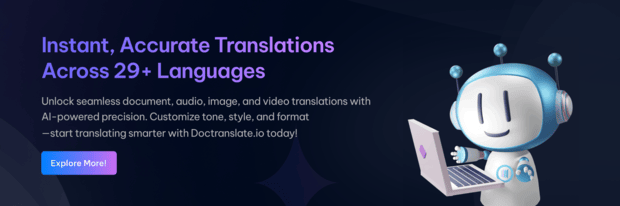
Để lại bình luận